The Limits of AI in Digital Vision: A Human Perspective
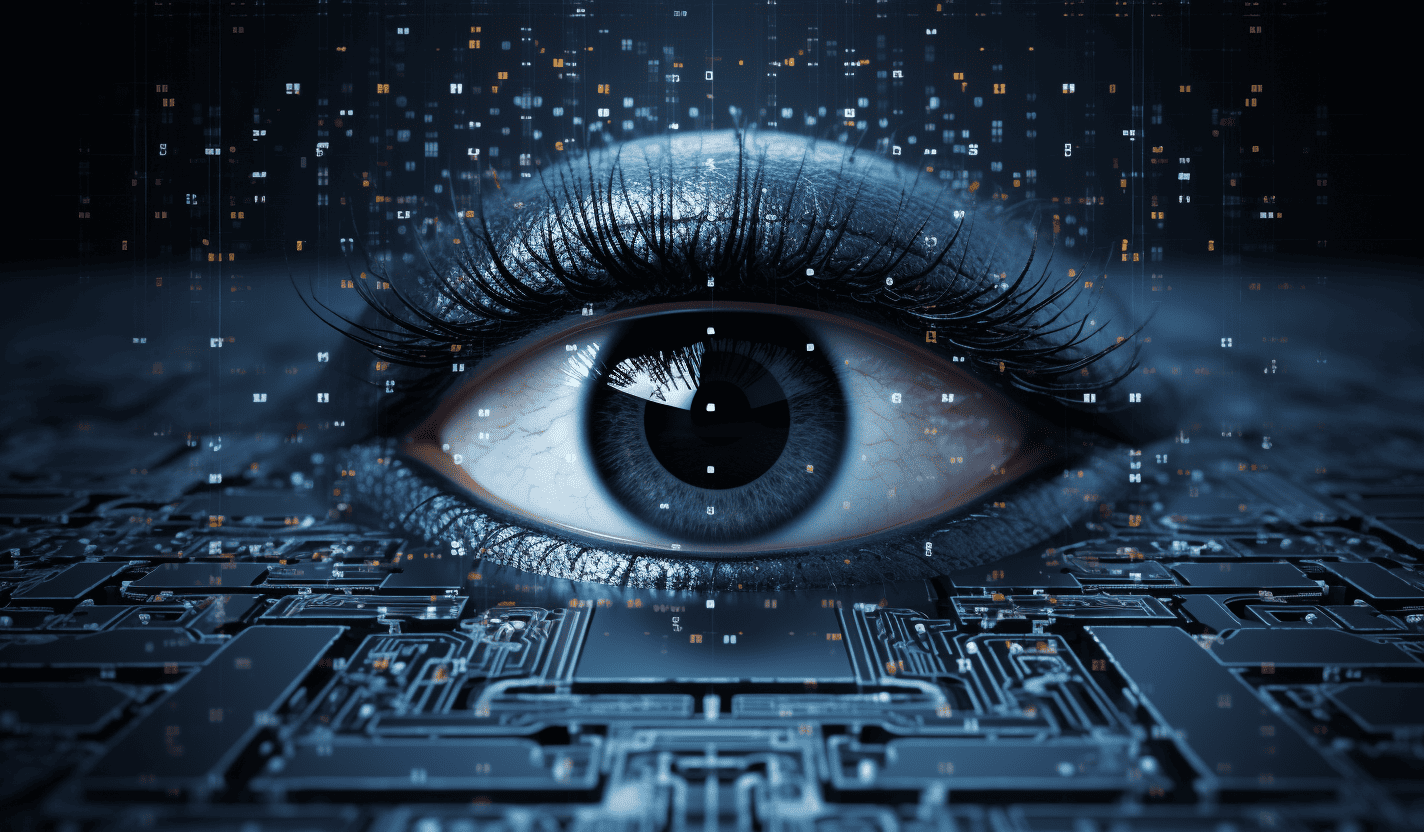
In the digital age, artificial intelligence (AI) has woven itself into our everyday lives, influencing everything from handheld devices to household appliances. A significant growth area for AI has been machine vision, a vital component of the digital vision concept. Digital Vision, capitalized on AI, has revolutionized numerous fields, including robotics, imaging technology, and autonomous driving. Yet, for all its progress, AI still needs to replicate the human visual system fully.
The challenges
Understanding the operation of digital cameras requires familiarization with certain technical concepts. ‘Rolling shutter‘ and ‘Global shutter’ are two of these. The rolling shutter method, which captures images line by line, often introduces distortions if the objects move rapidly during the capture. The global shutter method captures the entire scene simultaneously, avoiding such distortions.
However, when it comes to machine vision AI, these rolling shutter distortions can significantly impact the interpretation of an image. They present a challenge for AI, leading to complications in reliable and accurate image recognition tasks. Moreover, flickering, ghosting, and glowing phenomena add distortions and artifacts that can confuse machine-learning tools.
Another technique widely used in digital vision systems to improve image quality is High Dynamic Range (HDR) imaging. This method involves capturing and combining several images taken at different exposure levels to create a single photo with an enhanced range of colors and brightness levels. While HDR can produce stunningly detailed and vibrant images in static scenes, it can introduce its challenges when the subject moves.
Creating an HDR image involves taking multiple shots in quick succession. The resulting HDR image can contain artifacts when subjects move during this process. These inconsistencies occur because different parts of the image are captured at slightly different times. For
static scenes are not a problem, but these artifacts can introduce additional distortions and inaccuracies for dynamic scenes.
These artifacts can confuse AI algorithms when these HDR images are fed into machine learning tools. In trying to interpret the HDR image, the machine vision AI system can struggle to correctly identify and classify objects that appear distorted or smeared due to movement.
The Human Eye
Again, this is another instance where the human vision system proves to be a superior model. Our eyes and brain naturally adjust to a wide range of light levels in real-time, effectively performing an organic form of HDR without introducing motion artifacts. As we continue to develop machine vision AI and other artificial intelligence in robotics applications, these challenges provide us with areas to focus our efforts on. While rolling shutter and HDR introduce their challenges, they aren’t the only obstacles for standard sensor + ML systems. For instance, in the context of LED panels, flickering occurs due to the refresh rates of the panels. When the sensor’s refresh rate coincides with the LED panel’s refresh rate, the panel might appear off in the captured image. Ghosting, which leaves behind traces of moving objects in a sequence of frames and glowing, usually resulting from overexposure, further distorts the image data. These artifacts contribute to the inaccuracies in AI algorithm output, complicating the task of reliable image recognition and interpretation.
Looking for a solutions
Redundant sensors are sometimes included in the vision system to address the issues discussed in the previous section. Although these extra sensors can offer multiple perspectives and additional data, they also increase complexity and cost, which can lower the overall system reliability. Additionally, these alternative solutions often require significant computational power, and their effectiveness can vary, particularly when dealing with images that involve fast motion.In contrast, the human visual system, which is the original “digital eye,” can swiftly adjust and process visual data. It can handle distortions and artifacts without any noticeable effort. Our natural eyes form a part of an evolved system of biological AI that can process complicated visual data in real time. Despite the advancements in artificial intelligence in various fields, like robotics and autonomous driving, these challenges demonstrate the adaptability and resilience of the human visual system. Machine learning tools are yet to match the capabilities of our natural eyes. We are still on the path to developing and enhancing AI’s digital vision. With the promise of a future where AI might one day match or even surpass the complexity of human sight.
A revolutionary leap forward
While the challenges in digital vision seem daunting, solutions are emerging that aim to bridge the gap between machine vision AI and human visual capabilities. Our company, EYE2DRIVE, has developed a sensor that mimics the functioning of the human eye.
Our sensor technology is naturally immune to problems like HDR-induced artifacts, flickering from LED panel refresh rates, and ghosting. By capturing and processing images more like a human eye, our sensor offers a level of adaptability and reliability that traditional machine vision systems struggle to achieve.
Are you interested in learning how EYE2DRIVE can revolutionize your AI-driven imaging needs? Contact us for more information and take the first step towards next-generation digital vision solutions.