Expert Opinion: Francesco Lacapra & the Uncharted Terrains of Autonomous Driving
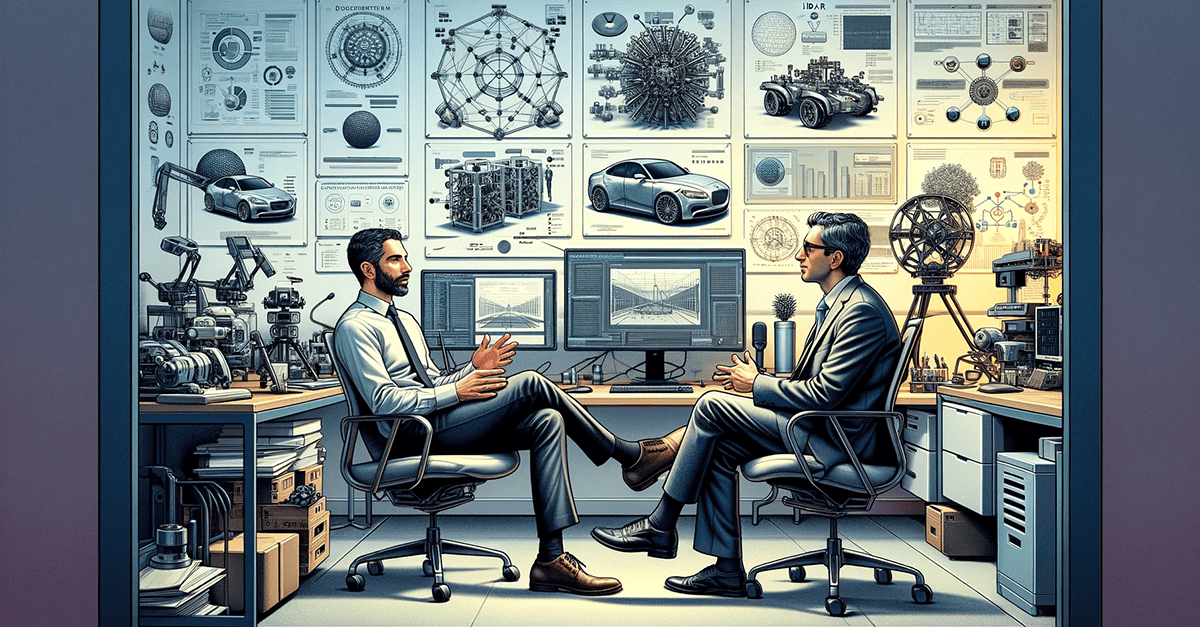
Francesco Lacapra stands out as a leading figure in the world of Autonomous Driving (AD) technology and its associated sensors for navigation. Originally from Italy, Francesco left his career at Olivetti and Enel to move to California, where he has worked ever since as an electronic engineer and a distinguished computer scientist.
Francesco is a skilled professional with a unique combination of technical expertise and innovative thinking. He has made valuable contributions to the development of autonomous vehicles in Silicon Valley. In this interview, we will leverage his skills to analyze trends and future developments for the advanced sensors required for autonomous driving navigation. Francesco’s expertise extends beyond his engineering skills. He also has a deep understanding of how software architecture impacts the systems that drive these vehicles. This comprehensive knowledge allows him to integrate complex sensor technologies seamlessly with sophisticated algorithms for autonomous navigation. Francesco Lacapra’s work on autonomous driving has consistently pushed the boundaries of what’s possible in this technology. He is a vital asset in the ever-evolving landscape of autonomous driving and related technologies.
1. Francesco, please share some information about yourself and your professional activities.
My career was initially focused on Operating System Design and Distributed Systems within Olivetti. I was also a professor in the Computer Science Department at the University of Milan for 8 years. I have founded a couple of startups focused on highly scalable distributed file systems. In the last eight years, I have worked on the development of software for Autonomous Driving (AD). My main areas of interest are software architecture and design, distributed systems, and AD infrastructures.
2. What excites you the most in your daily job?
These days, there are very well-trained vision and control engineers with advanced degrees who work in AD. They generally excel in the development of very high-quality and effective algorithms to perform environment perception and control vehicles. However, sometimes their background does not include a deep understanding of software technology and software architecture. This is a challenge.
Coupling the ability to implement sophisticated algorithms for localization, perception, and actual vehicle motion planning with well-engineered software layering and infrastructure leads to better and more predictable performance and more general solutions. I am very focused on this space, where bridging such different disciplines can lead to delivering better and more robust products for this rapidly emerging market. Of course, I also enjoy spending a fair amount of my time learning about new sensors and ancillary technologies that can sooner or later allow the entire AD field to grow and improve.
3. Among the technologies you work with daily, directly or indirectly, which one still hasn’t expressed its full potential?
From the sensor domain, one technology that, in my opinion, still has enormous potential and yet is not yet able to reach a reasonable compromise among three different attributes (cost-effectiveness, capabilities, and long-term fungibility) is the LiDAR technology. A LiDAR’s ability to clearly identify the distance and possibly the velocity of surrounding objects is key. However, this is generally done via electromechanical devices that lack long-term reliability, may need frequent adjustments, and are expensive. Technologies that have been proposed for years, based on electronic steering of laser beams via phased-array approaches, are still not viable, especially in terms of low-cost products. In addition to this, the inherent inability of LiDARs to identify color attributes of surrounding objects still requires cameras to be present.
Cameras have made incredible progress. Still, being able to detect objects in poor lighting conditions is a problem. Moreover, the range of detection is often limited, such that applying it to vehicles that may move at high speed can create concrete risks of not being able to perceive obstacles sufficiently ahead of time.
Finally, software fusion technology that must be used to provide a unified view of individual objects using LiDARs, cameras, and radars is still complex to put together in many cases.
4. Eye2Drive is working on the imaging sensor technology, leaving it to the integrators to develop the AI controller. Based on your experience, what are the benefits and limitations of our approach to Autonomous Driving vision?
I have mostly worked with OEMs (vehicle manufacturers). What is needed from the OEM’s point of view is a finished product rather than a building block to be made part of a full solution. The difficult issue is that as OEMs are moving towards AD systems, they have started to understand that including an AD system developed externally by a system integrator into their vehicles is likely not going to work. Despite some of their shortcomings, at Tesla, it has been shown that vehicles that want to cover the AD space need to be designed as wheels surrounding a computer rather than as vehicles with a computer attached. Effectiveness and ability to support well-integrated AD systems require the AD software to be developed closely with the sensor infrastructure.
To what extent could an externally-supplied AI controller be able to integrate successfully with the vehicle software? Would, in turn, the OEM be forced to develop also the integration software? What kind of additional problems common to existing camera sensors would a new design such as the one you are mentioning be able to solve because of its specific technology strengths? Would the additional investment an OEM would need to develop the integration software provide a significant ROI? If the approach used and the intrinsic attributes of this new type of camera sensor are really different, what guarantees would an OEM have that the company and the technology would not disappear because of lack of traction and funding?
5. What significant challenges prevent AD’s widespread implementation and adoption? When do you think they will become accessible to the general public?
I work in AD for a segment where the technology is already good enough. We are talking about autonomous vehicles operating in relatively small areas that are not open to the public and have very minor human presence. This simplifies the problem greatly because localization can always be accurate, also through the use of markers, beacons, and whatnot. Moreover, the safety requirements are much more limited than they would be in the more general case.
In the area of “hub-to-hub” AD (consisting of vehicles that move over freeways between end stations), the technology is close to where it should be, and I would expect it to be fairly fungible for significant deployment within 3 to 5 years.
In the case of general-purpose driving, including urban driving and so on, it is just difficult to deal with all the complex cases that would hinder reasonable probabilities of success. Some of the technologies in use are not scalable, like the ones heavily dependent on extremely accurate high-definition mapping of the areas where a vehicle must be able to drive.
First of all, very accurate mapping requires continuous deployment of mapping vehicles, and this is not feasible on a large scale and with the fine granularity needed. Secondly, a good general-purpose AD solution, in my opinion, should be able to operate correctly by avoiding critical issues regardless of the area or of how the situation may appear different from the expectations due to random events. I do not believe that a fully usable and scalable solution will be available for level 4 AD before eight years or so.
6. Several channels feed the navigation system with data, including digital maps, machine learning algorithms, and sensors. What role does each of these sources play in the final driving decision?
As I pointed out in my previous reply, some of the solutions being deployed (think of Waymo) are too dependent on the high accuracy of fine-grain maps. I believe that machine learning, sensors, and algorithms must be the ones that do the heavy lifting. As I mentioned earlier, I believe there are current limitations with sensors and algorithms. I also believe that contrary to some existing opinions, relying mostly on machine learning is a problem. Machine learning has a role in certain problem areas but should have none in others.
For example, the physics of a vehicle is perfectly known. There are algorithmic solutions to perform drive planning based on velocity, acceleration, weight, and other vehicle parameters. Relying on machine learning for this is a major mistake. On top of that, there is also the issue that perception must rely on machine learning. Machine learning-based solutions can only be statistically validated, whereas it is impossible to offer axiomatic proof of correctness. This has an impact on the validity of the solution and the legal liabilities deriving from it.
7. The investment in autonomous driving technologies is impressive. Do you expect any significant spillover with these technologies applied to other areas?
Most definitely. I believe that many of the steps forward in many more limited areas offer definite deployment opportunities. Think of industrial automation, warehouse management, vehicle loading and unloading. Even flying machines can benefit much more from the progress being made. This area has the significant benefit of being able to rely on a 3D space (rather than a 2D one), where one extra degree of liberty improves things in terms of maneuvering. Of course, the flight dynamics are more complex, but it is also a matter that has already been solved.
Conclusion
We thank Francesco Lacapra for sharing his invaluable insights and expertise in Autonomous Driving technology and its critical sensor technologies. His depth of knowledge and experience in this field offers a unique perspective on the challenges and future directions of AV and related technologies.
As we continue to explore these dynamic and rapidly evolving technological landscapes, we invite our readers to join the conversation. Your opinions, questions, and contributions are welcome and essential in fostering a diverse and enriching discussion. Whether you’re a professional in the field, a technology enthusiast, or simply curious about the future of autonomous driving, we encourage you to share your thoughts and ideas in the comments section below. Let’s delve deeper into these important topics and learn from each other’s perspectives.
Very insightful article from a domain expert with a deep understanding of the space. Thank you for posting this.
Thanks Raffaello! Francesco is a real expert!